
Intelligent Products
AI challenges: Tackling 2 key hurdles in building Intelligent Products
Developing and launching Intelligent Products is a completely new experience for many companies that haven’t yet employed AI/ML technology to create business value. The complex and cutting-edge nature of the work only adds to the learning curve. Knowing the AI challenges ahead of time means you can prep your team and work through or even avoid some of the stickier problems.
Here’s a quick reminder of why companies choose to build intelligent products:
- To generate business value. Creating AI/ML solutions (not just prototypes) is what requires teams to operate and collaborate in new ways, and it’s also what drives value.
- To increase competitive advantage. For example, you can get to market faster, deliver superior support, or deliver unique innovative products.
- To provide beneficial experiences for customers and meaningful work for employees.
If you’re a business leader considering intelligent products for your roadmap or an engineer gearing up for a project, here’s some advice on how to succeed from our clients and Builders who’ve been there.
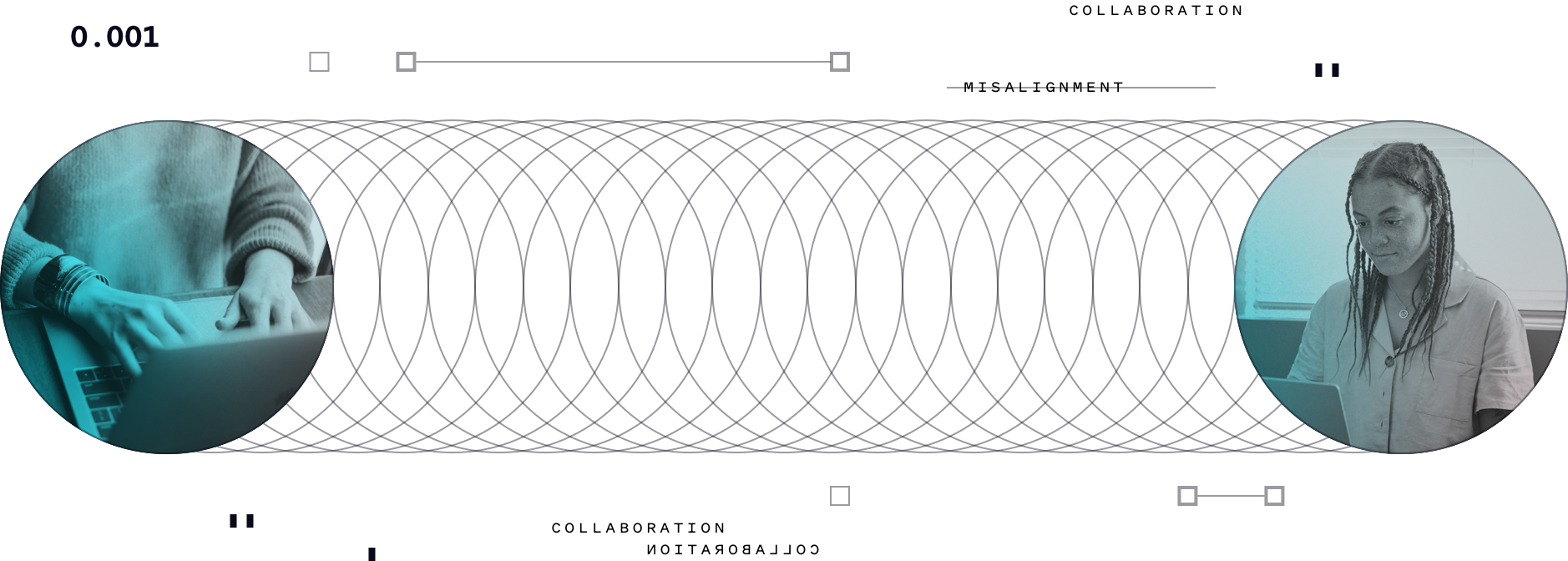
AI challenge no. 1: Getting different groups to collaborate
Companies have good reasons for the way they are organized. Functional groups have independence so they can prioritize and perform the work requested of them. They follow specialized processes and best practices suited to their discipline.
Some divisions are inherent rather than intentional. Teams around the world work in various time zones and speak different languages, and their workstyles are influenced by their cultural dynamics. C-suite executives and other business leaders are busy running the organization and don’t have day-to-day contact with the technical experts who bring product plans to life.
Building intelligent products requires that people work across many of these silos in significant ways. And that’s where the difficulties start to arise.
Misalignment can lead to wasted effort.
C-suite executive: “Let’s make this cool disruptive product, X.”
Engineer: “Here you go, we built Y.”
Customer: “Sorry, I only want to buy Z.”
Contributors to one aspect of the project make assumptions that don’t account for other aspects. When the conflicts are discovered, it’s not clear how to adjust.
Software engineer: “You need to fix the data you send us.”
Data engineer: “No, you need to rebuild your thing to work with what we sent you.”
How to improve cross-capability collaboration
- Begin with an alignment phase, when technical experts and business leaders discuss different options and key considerations to help balance feasibility, budget, and timeline. During this phase, do market research to make sure that customers want what’s being built and that the investment is worth it.
- Have a representative from each capability play a role on the product team. This structure provides a direct connection with the project and ownership of the outcome.
- Enable daily interaction between the project team and the business stakeholders. The more frequent the interaction, the less likely a divergence in work and priorities.
- Protect the business hours that overlap between groups so people can spend as much of that time as possible working together.
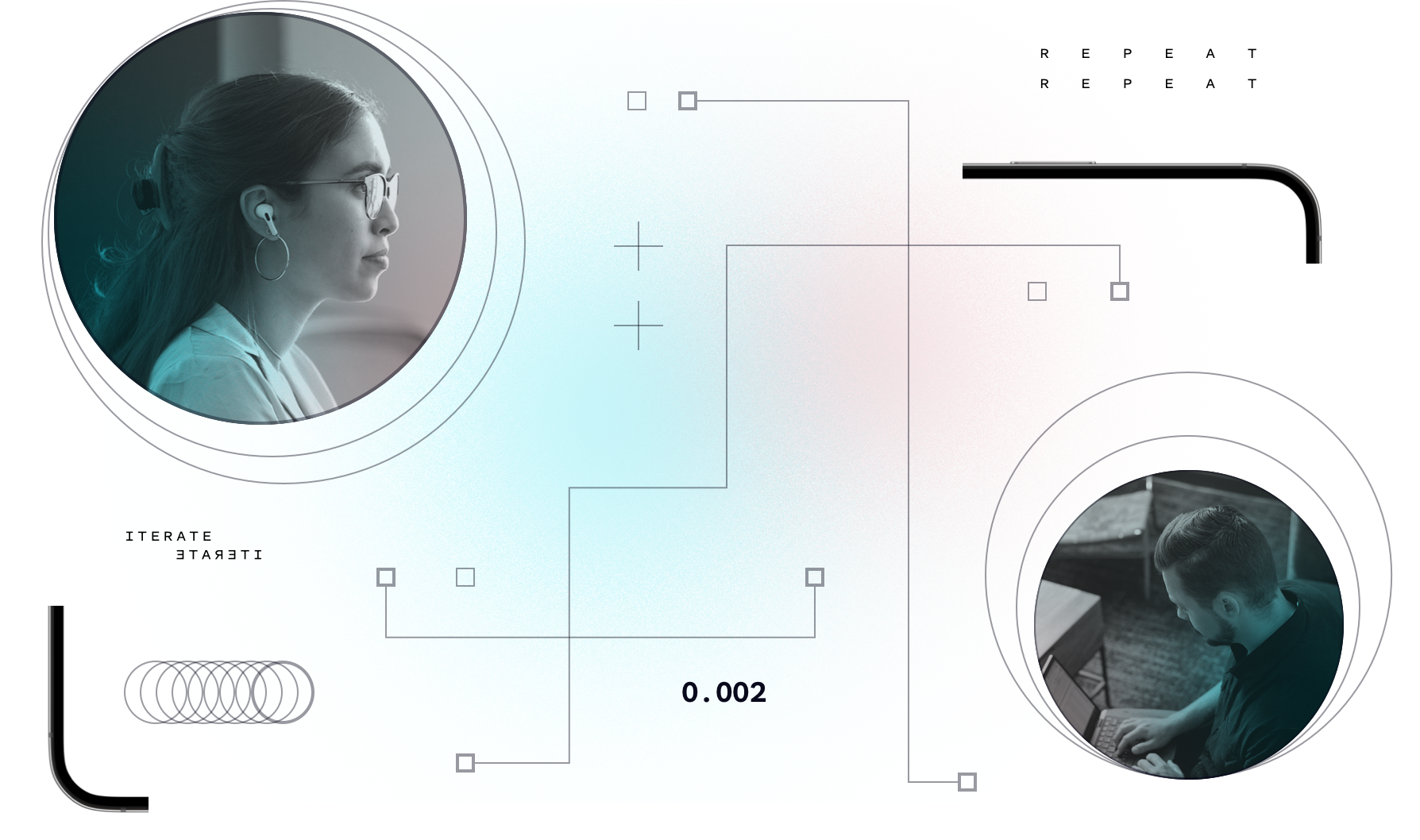
AI challenge no. 2: Learning new approaches to operations
Intelligent products go beyond the proof-of-concept stage to full market offerings. So, the scaling and iteration required to produce a commercial product means that team members must adapt their way of working—especially machine learning practitioners.
Data science teams are often used to working in a waterfall manner, even if the work is managed through scrum sprints. When the data science team is separate from the software engineering team and the MLOps team, they hand off code that hasn’t been integrated with the software and doesn’t follow MLOps practices.
Data scientist: “Here’s the code. Okay, I’m done, see you later.”
Software engineer: “Wait, what is this? I’ve never seen a Jupiter notebook before.”
Historically, releases of new data science models are painful experiences. So, ML practitioners naturally want to minimize the number of releases, which makes them even more complex. Chasing near-perfection in data science work creates delays and integration challenges further down the line.
Data scientist: “What’s the best model we can train to solve this machine learning problem?”
Solution owner: “How about let’s start with, what’s the first thing we can do to solve this business problem?”
How to adapt operations for Intelligent Products
- Employ Agile methods rather than just doing code hand-offs. Code should be released early to a development environment where team members can all start iterating on it. When the time comes for the code to be released, it already incorporates all the different components needed, and the release will be a much smoother process.
- Embrace MVPs (minimum viable products). Identify what “good enough” looks like out of all the possibilities for the intelligent product. Define your MVP through workshops before the project starts and refine it during the initial discovery phase.
- Teams should be working together closely to continuously adapt the product to meet the desired experiences as they evolve. Instead of shipping once and then doing bug fixes forever, ship and then iterate. Take the insights gained and operationalize them.
Making changes to meet the demands of intelligent products is a unique kind of “transformation by doing” for leading organizations.
As all of Slalom, we’re uniquely positioned to come in and solve these hybrid technical-plus-organizational challenges. So we’re asking, how can we set our clients up to be successful when the challenge is not just technical, and it’s not just organizational, but it’s really both going hand in hand?
Miles Erickson, Principal, Machine Learning & Data Engineering, Slalom Build
To learn more about AI challenges and the rewards of developing intelligent products, check out our whitepaper, Building Your Future with Intelligent Products.